.avif)
Explaining complexity in research: Embracing new methods
Being a market researcher sometimes entails explaining complex research outputs to first-time clients who then have to retell the insights to internal stakeholders. However, for behavioral researchers, clarifying complex results to puzzled clients who are new to implicit research is a part of the day-to-day work. We compiled useful advice, Do’s, and Don’ts to help corporate researchers make sense of the thrilling world of implicit insights. This is the second part of a two-part conversation between 4 Insights directors and managers at EyeSee – read part 1 here.
What convinces people to add new methods to their insights arsenal?
Sanja Cickaric: When it comes to persuading the stakeholders to try behavioral testing instead of traditional research, two great reasons usually turn them into believers. Even though virtual shopping is not identical to shopping in front of a real shelf, and a task-based e-commerce study is not the same as real shopping online, implicit research is, simply put, “cleaner” than self-reported data. We don’t rely on the plethora of biases that are at play in self-reporting. Secondly, we use a monadic design and A/B testing in all our studies (i.e., multiple cells that we can compare) that is much more reliable than comparing our results to offline, standard research techniques. This would be the market research equivalent of comparing apples to oranges.
Another thing our clients need help with is understanding visibility data; what number is a good number when it comes to visibility? Here again, this needs to be solved by an A/B design, or by introducing a benchmark value to compare the results to."Implicit research is, simply put, “cleaner” than self-reported data", Sanja Čičkarić, Digital insights director
Are insights people less prone to a rigid mindset?
Marija Smudja: It’s not only the stakeholders that have trouble with mixed results – sometimes the researchers do, too. For a complete analysis of contradicting implicit and explicit measurements, we need to use a holistic approach and always look for the reasons why. For example, we can have an ad with high emotional engagement, with an excellent holding power in the environment – great results on implicit measurements – but at the same time, have very low likability and brand fit. On the surface, these are opposing KPIs. However, with more in-depth analysis, we might learn that they are not opposing at all. The ad is triggering a high level of emotions, sure, but those are mostly difficult or negative emotions (disgust, fear, sadness), so that would explain low likability. So, it is not enough to take a quick look at the KPIs and check if the implicit and explicit match, but look for the reasons. Only when we understand what each specific KPI is telling us, we can paint the whole picture."It is not enough to take a quick look at the KPIs and check if the implicit and explicit match, but look for the reasons [why]" Marija Smuđa, Advertising insights director
Direct preference choice and its perils
Dobrinka Vicentijevic: There is another piece of advice I would add: make the study design clear upfront, because it will simplify your life and conversations down the way. Also, use a monadic design. What sometimes happens though, is that in a monadic study, at the end of our survey/test, we sometimes get results that are confusing for the clients. For example, if we test 3 new packaging designs, and want to compare them with the existing packaging, we will have 4 cells so that one respondent only sees one of the new designs throughout the test. However, sometimes clients decide to put the respondents in an unnatural situation: have them compare the packaging they were exposed to for the entire duration of the test with the existing packaging and say which one they prefer, in a so-called forced-choice situation.
This is done in case the resulting data doesn’t tip over in favor of any of the proposed new designs, in order to gain at least some insight regarding the efficiency of proposed new designs. So, what is the issue with this? For example, the winning design is Design 1 on all of the most important KPIs, but in a direct preference choice, Design 1 loses from the Current design – which fared worse on all key KPIs and criteria. This confuses the clients immensely. In such situations, we try to explain that this particular bit of info is not as valuable as the rest of the data, because it is not a realistic situation for the respondent. People will never see two pack designs of the same product on the shelf and have to choose between them. Additionally, in cases in which people face a choice, they are more inclined to pick less risky options (i.e., the old design, which is safer, and more familiar to them)."[When] people face a choice, they are more inclined to pick less risky options", Dobrinka Vićentijević, Shopper insights director
Researchers have a responsibility of making complexity manageable
Even though trying out implicit research might be a big step for researchers used to conventional research, particularly if they have substantial historical databases and years of data to lean on, it is worth finding a partner you can rely on to clarify any issues along the way, and get the opportunity to increase the predictive power of your research with the added value of a new complementing dataset.
Marija Smuđa, Advertising insights director, EyeSee
Dobrinka Vicentijevic, Shopper insights director, EyeSee
Sanja Cickaric, Digital insights director, EyeSee
Reckitt x EyeSee
@ESOMAR Retail Media webinar on demand
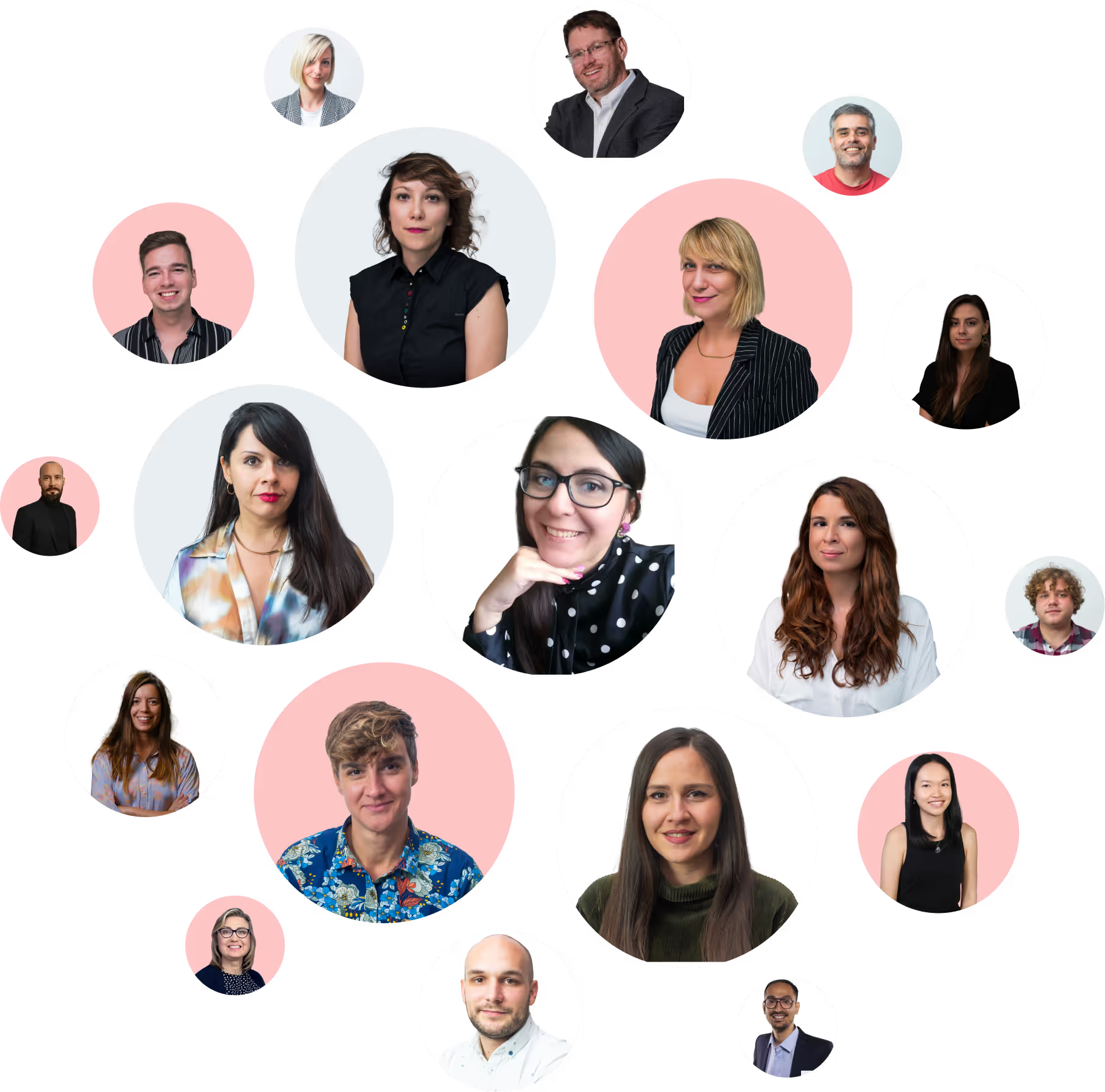