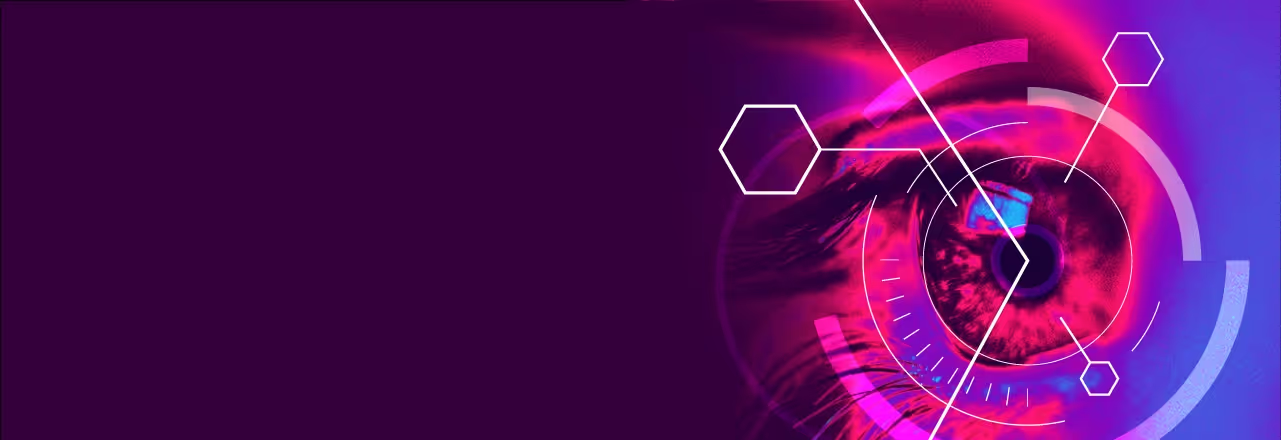
Know when to play the AI card in eye tracking
Facing high inflation, most decision makers in market research are trying to find alternatives for quick savings. For example, AI-based visual prediction models promise to eliminate the need for eye-trackers as they give faster and more affordable results; and supposedly - just as good.
Let’s examine if this is really the case.
BTS of human gaze analyses
While both AI predictive algorithms and eye trackers seek to provide human gaze estimations and help us understand attention and visibility KPIs, they operate fundamentally differently.
Regular eye trackers rely on the data obtained from mapping the respondents’ eye gaze, while saliency-based AI solutions predict behavior based on the audio-visual features of the stimuli gazed at, relying on insights from cognitive science – what we know about how humans usually perceive things. In essence, this means that regular eye trackers deliver an answer to what is seen, while AI models suggest what is probable to be seen.
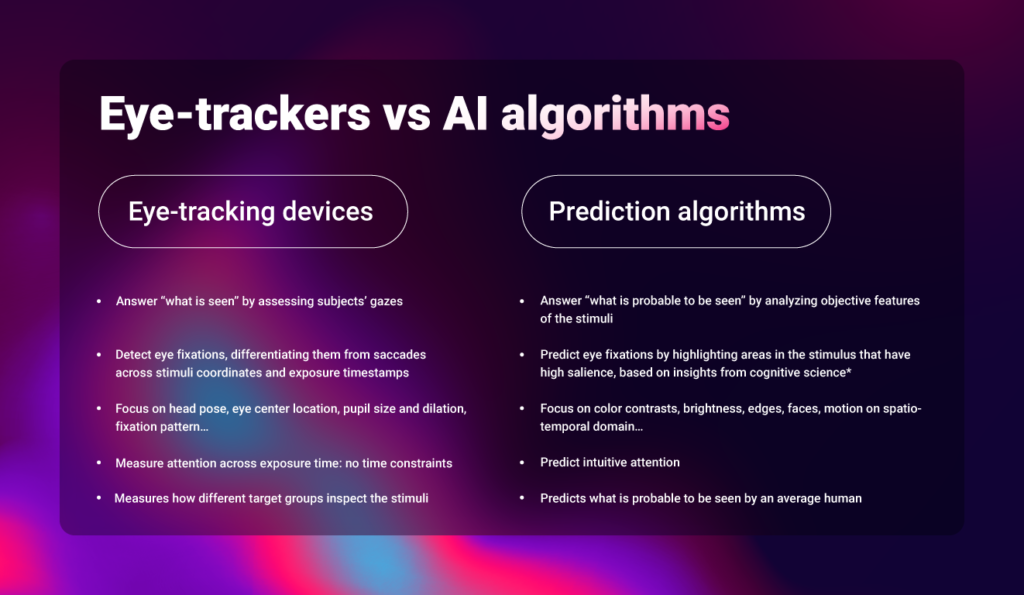
Consequently, eye trackers can chart the variance in behavior of different target groups inspecting stimuli, while prediction algorithms showcase the probability of what is seen by an average human. This means that if your study is looking at an average population, or you are looking for a simple reality check of early development concepts – AI might be good enough for the job. For all other cases, validation or full application of eye trackers is still advised.
Scientific and practical gaps
When talking to an AI vendor, it's important to ask about the timeframe for which their model predicts visibility. Does it predict what's most likely to be seen on a product packaging or a webpage at first glance, or does it predict visibility after the user has browsed for some time? You may be surprised by the variety of answers aka absence of any standard in the AI field, as well as by the inconsistency of some models with what we know from cognitive psychology about human memory.
Some AI solutions predict intuitive attention and visibility in the first 150 – 250ms. According to the Atkinson-Shiffrin memory model, when we see something, the entire information registered by our eyes is first stored in our sensory memory. This is a very short-term storage that only lasts for about 250 milliseconds, during which time the information is not yet processed in any way. Only when the information passes into the working memory, under the effect of selective attention, is the limited information about the stimuli being processed but for no longer than 15 seconds (with the average human attention span tending to decrease towards 8 seconds). Eye-tracking devices usually capture attention ranging from 3 to 7 seconds, coinciding with the duration of information processing. Going beyond theory, even from a practical perspective, is it really useful to know what can be observed on a website within a duration of 150-250 milliseconds?

Saliency is (still) mostly context-unaware
To fully understand the power and limits of the AI approach, my team recently completed a validation study of saliency-based algorithm for predictive eye tracking. We selected a number of stimuli typically tested in consumer research studies across industries and channels: from vertical and horizontal packaging stimuli to shelf images and webpages. We compared the results obtained by two different AI models available and popular on the research market with the results obtained using eye-trackers on human respondents.
What we’ve found is that saliency models are consistently under-predicting semantically important image regions; in some cases, they failed to catch the importance of a brand name on a pack aka failed to determine that those are not just any letters written in certain color, font and size.
What’s more, different models tend to favor different properties such as text, colors, faces, and others. Knowing that helps identify a model’s deficiency and choose an appropriate model for a specific application. For example, some models are better at capturing text area, while in some others, the sound is not considered. Further, different algorithms might not be trained on a certain type of stimuli, which could undermine findings for an entire category.
While this might get better with time, the overall conclusion is that more complex (full shelves, e-commerce websites) and dynamic (video ads) stimuli prove to be way too challenging for AI predictive algorithms as they are still incapable of contextual interpretation. They inspect the size and contrast, not the content; and in doing so, they often fail to capture the meaning. Knowing just how much meaning we attach to brands, letting AI conclude that it is looking at a purple cow feels stripped of all the meaning we attach to a brand like Milka.
An excerpt from a recent study published by the Institute of Electrical and Electronics Engineers provides an additional point of comparison between prediction algorithms and real eye-tracking.
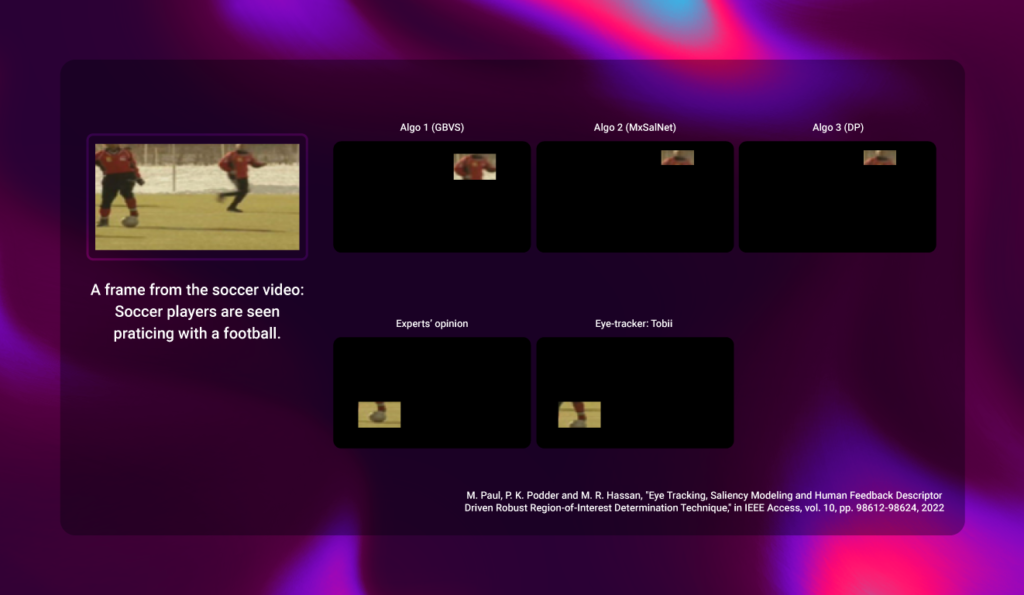
Three different algorithms were used to predict what humans would look at while watching soccer players in colorful jerseys practicing with a football. The first algorithm picked up the colored regions as the most significant points, while the other two algorithms were concentrating on the players’ faces. Both underlying assumptions are justified from a cognitive science point of view: colors (especially red), and faces do grab people’s attention. However, true eye-tracking data showed that people were looking at the ball during soccer match, because they understand the meaning behind this game.
Conclusion
So, what’s the verdict on AI in this case? We’ve previously established that not all AI predictive models are created equal; they process and favor different properties. Keep this in mind, when selecting for a solution - you might find yourself short of one single perfect algorithm; in reality, you’ll have to choose every time you conduct a study.
However, this does not erase the fact that predictive algorithms are more scalable than full eye trackers. It is possible to imagine a scenario where a single application gathers data about both the user and the stimuli. By leveraging the complementary nature of subjective human eye-tracking and objective graph-based visual saliency modelling data, such solution would ultimately lead to a more reliable and efficient output. Until then – real eye tracking is still supreme when faced with complex questions that require nuanced insights.
Interested in application of behavioral methods? Check out our previous blog on measuring attention!
Kellanova x EyeSee
@Quirk's Pack Redesign webinar on demand
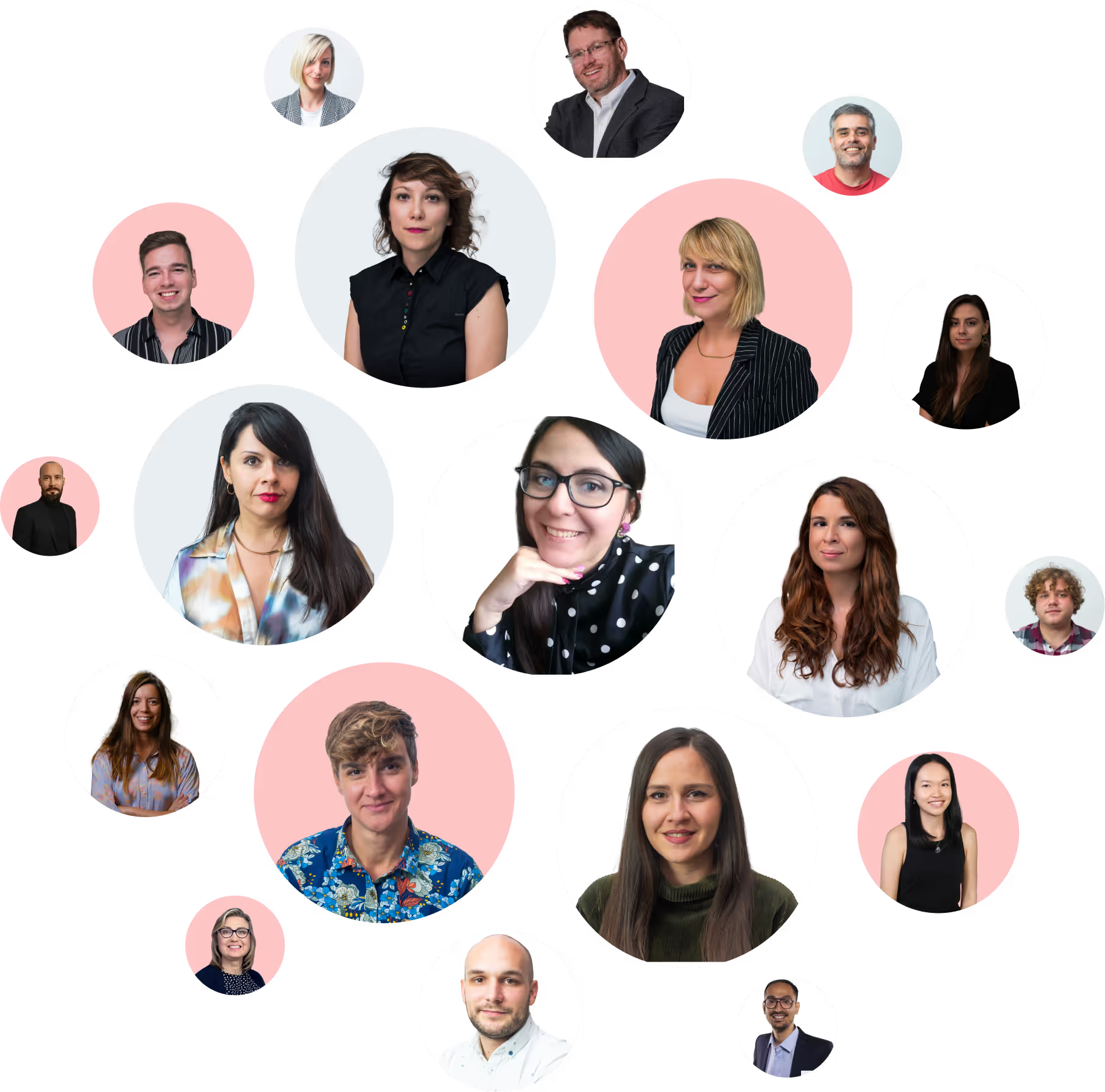